
Table of Contents
What is Generative BI?
Generative Business Intelligence (BI) refers to a cutting-edge approach in data analytics that focuses on not only providing insights into past and present data but also on generating actionable insights and predictions for the future. Unlike traditional BI, which primarily focuses on reporting and analyzing historical data, generative BI leverages advanced machine learning and artificial intelligence techniques to generate new data points, scenarios, or predictions based on existing data.
This proactive approach enables businesses to anticipate trends, identify opportunities, and mitigate risks more effectively. Generative BI tools can automatically generate various scenarios and simulations, allowing businesses to make data-driven decisions with greater confidence and agility. By harnessing the power of generative BI, organizations can gain a competitive edge in today’s rapidly evolving business landscape by unlocking new insights and opportunities that drive innovation and growth.
Introduction to Generative BI
Generative Business Intelligence (BI) represents a paradigm shift in the realm of data analytics, poised at the intersection of predictive modeling and traditional BI practices. Unlike conventional BI methods that predominantly focus on historical data analysis and reporting, generative BI extends its reach to forecast future trends, behaviors, and outcomes.
This innovative approach harnesses the capabilities of advanced machine learning algorithms and artificial intelligence techniques to generate actionable insights and predictive models. By extrapolating patterns from existing data sets, generative BI empowers organizations to anticipate market shifts, identify emerging opportunities, and proactively mitigate potential risks. This introduction lays the groundwork for exploring how generative BI augments decision-making processes by furnishing stakeholders with foresight and agility in navigating complex business landscapes.
Understanding Business Intelligence (BI)
Business Intelligence (BI) is a comprehensive process that involves the collection, analysis, interpretation, and presentation of data to support decision-making within an organization. At its core, BI seeks to transform raw data into meaningful insights that drive strategic and operational improvements. The primary objective of BI is to enable stakeholders to make informed decisions by providing them with timely and relevant information. This information can range from simple operational metrics to complex predictive analytics, depending on the needs and goals of the organization.
Central to the BI process is the gathering of data from various internal and external sources. This data may include transactional records, customer interactions, market trends, and more. Once collected, the data is then processed and transformed into a format that is conducive to analysis. This often involves cleaning and organizing the data to ensure its accuracy and consistency.
Once the data is prepared, it undergoes analysis using various analytical techniques and tools. These analyses can range from simple descriptive statistics to more advanced data mining and machine learning algorithms. The goal of analysis is to uncover patterns, trends, and relationships within the data that can inform decision-making.
Interpretation of the analyzed data is a crucial step in the BI process. This involves extracting insights from the data and translating them into actionable recommendations for stakeholders. Effective interpretation requires a deep understanding of the business context and domain expertise to ensure that the insights generated are relevant and meaningful.
Finally, BI involves the presentation of insights in a format that is accessible and understandable to stakeholders. This often includes the creation of reports, dashboards, and visualizations that communicate key findings and metrics. By presenting information in a clear and intuitive manner, BI helps stakeholders quickly grasp the implications of the data and make informed decisions.

What Sets Generative BI Apart?
What sets Generative Business Intelligence (BI) apart is its forward-looking nature and its utilization of advanced machine learning and artificial intelligence techniques. Unlike traditional BI, which primarily focuses on analyzing historical data to understand past trends and behaviors, generative BI extends its capabilities to predict future outcomes and generate new data points. This proactive approach allows organizations to anticipate market shifts, identify emerging opportunities, and mitigate risks before they fully materialize.
Generative BI harnesses the power of algorithms to simulate various scenarios and predict potential outcomes based on existing data. By extrapolating patterns and trends, generative BI enables organizations to make informed decisions and develop proactive strategies to stay ahead of the curve. This contrasts with traditional BI, which often relies on reactive analysis of past data to inform decision-making.
Another distinguishing feature of generative BI is its ability to automate the generation of insights and predictions. Through the use of machine learning models, generative BI systems can continuously analyze data and adapt to changing circumstances in real-time. This automation not only enhances the speed and efficiency of decision-making but also frees up valuable human resources to focus on more strategic tasks.
Overall, what sets Generative BI apart is its focus on predictive analytics, automation, and agility. By combining advanced analytical techniques with proactive decision-making, generative BI empowers organizations to navigate uncertainty, seize opportunities, and drive innovation in today’s dynamic business environment.
Key Components of Generative BI
Machine Learning Algorithms
At the core of generative BI lie sophisticated machine learning algorithms. These algorithms analyze historical data, identify patterns, and generate predictive models to anticipate future trends. By continuously learning from new data inputs, machine learning algorithms adapt and refine their predictions, enabling organizations to make proactive decisions.
Data Integration
Generative BI relies on seamless integration of disparate data sources, including structured and unstructured data. Data integration ensures a unified view of information across the organization, enabling comprehensive analysis and insightful decision-making. Advanced data integration techniques streamline the process of data ingestion, cleansing, and transformation, enhancing the accuracy and reliability of BI insights.
Predictive Analytics
Predictive analytics forms the cornerstone of generative BI, enabling organizations to forecast future outcomes with precision. By leveraging statistical algorithms and machine learning models, predictive analytics identifies patterns, trends, and correlations within data sets. These insights empower businesses to anticipate customer behavior, optimize resource allocation, and mitigate risks effectively.
Applications of Generative BI
Generative BI finds applications across various industries and functional areas, revolutionizing decision-making processes and driving strategic initiatives. Some prominent applications include:
Marketing Optimization
Generative BI enables marketers to gain deep insights into customer preferences, behavior, and purchasing patterns. By analyzing historical data and predicting future trends, marketers can personalize marketing campaigns, optimize advertising spend, and enhance customer engagement.
Financial Forecasting
In the realm of finance, generative BI facilitates accurate forecasting of financial performance, market trends, and investment opportunities. Financial institutions leverage predictive analytics to assess credit risk, detect fraudulent activities, and optimize portfolio management strategies.
Risk Management
Generative BI plays a pivotal role in risk management by identifying potential threats, vulnerabilities, and emerging trends. Through advanced analytics and predictive modeling, organizations can proactively mitigate risks, comply with regulatory requirements, and safeguard their assets and reputation.
Advantages of Generative BI
Generative BI offers several compelling advantages that drive its adoption and implementation across organizations:
Enhanced Decision Making
Enhanced decision-making refers to the process of improving and optimizing the choices made by individuals or organizations through the utilization of advanced tools, data, and insights. This enhancement can take various forms, such as increased accuracy, timeliness, and relevance of decisions. One key aspect of enhanced decision-making is the integration of data-driven insights, where organizations leverage data analytics and business intelligence to inform their decision-making processes. By analyzing large volumes of data, identifying patterns, and predicting outcomes, decision-makers can make more informed and strategic choices.
Moreover, enhanced decision-making often involves the incorporation of predictive analytics, allowing organizations to anticipate future trends and potential outcomes. By leveraging predictive models and algorithms, decision-makers can proactively address challenges and capitalize on opportunities before they arise. This forward-looking approach enables organizations to stay ahead of the curve and adapt to changing market conditions more effectively.
Additionally, enhanced decision-making may involve the use of automation and artificial intelligence technologies to streamline decision-making processes. By automating routine tasks and leveraging AI-driven insights, organizations can make faster, more accurate decisions while freeing up valuable human resources to focus on higher-value activities.
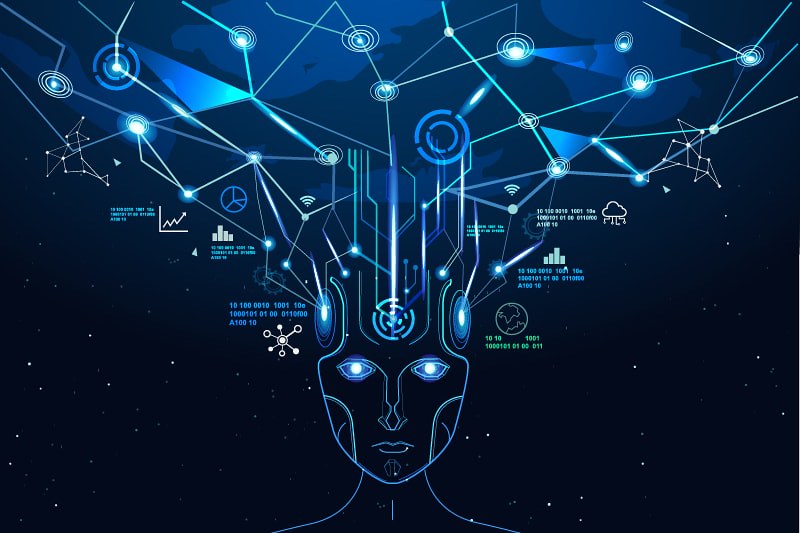
Real-time Insights
Generative BI facilitates real-time monitoring and analysis of key performance indicators (KPIs), enabling stakeholders to track business performance and detect anomalies promptly. Real-time insights empower organizations to seize opportunities and address challenges proactively.
Real-time insights refer to timely and up-to-date information derived from the analysis of data as it is generated or collected. These insights provide immediate visibility into current trends, patterns, and events, allowing organizations to make informed decisions quickly and effectively. Unlike traditional analytics, which often involve batch processing and retrospective analysis, real-time insights enable decision-makers to respond promptly to changing conditions and seize opportunities as they arise.
One key advantage of real-time insights is their ability to capture and analyze data streams as they occur, providing a dynamic and continuous view of business operations. This real-time visibility allows organizations to detect anomalies, identify emerging trends, and monitor key performance indicators (KPIs) in the moment. By staying abreast of developments in real-time, decision-makers can take proactive measures to address issues, optimize processes, and capitalize on opportunities without delay.
Moreover, real-time insights enable organizations to enhance agility and responsiveness in their decision-making processes. By accessing timely information, decision-makers can adapt strategies, allocate resources, and adjust tactics in real-time to meet evolving business needs and customer demands. This agility is particularly valuable in fast-paced industries where rapid decision-making can confer a competitive advantage.
Additionally, real-time insights facilitate collaboration and communication across different departments and teams within an organization. By providing a common, up-to-date view of data and metrics, real-time analytics foster transparency and alignment, enabling stakeholders to work together more effectively towards shared goals and objectives.
Scalability and Flexibility
Scalability and flexibility are two essential characteristics that contribute to the effectiveness and adaptability of systems, processes, or solutions within an organization. Scalability refers to the capability of a system to handle increasing workloads or accommodate growth without compromising performance or functionality. It involves the ability to seamlessly expand resources, such as computing power, storage capacity, or bandwidth, to meet evolving demands. Scalable solutions can easily accommodate changes in workload size or user base without requiring significant redesign or reconfiguration, making them well-suited for dynamic and growing environments.
Flexibility, on the other hand, refers to the degree to which a system or solution can adapt to changing requirements, preferences, or conditions. Flexible solutions are characterized by their ability to be easily customized, configured, or modified to suit specific needs or preferences. They offer users the freedom to adjust parameters, workflows, or functionalities to align with evolving business objectives or operational processes. Flexible solutions can support diverse use cases, accommodate variations in user preferences, and integrate seamlessly with existing systems or workflows, enhancing agility and responsiveness.
In combination, scalability and flexibility contribute to the robustness and versatility of systems and processes within an organization. Scalable and flexible solutions can grow and evolve alongside the organization, adapting to changing needs, expanding capabilities, and accommodating fluctuations in demand or usage patterns. By investing in scalable and flexible solutions, organizations can future-proof their operations, optimize resource utilization, and maintain a competitive edge in today’s rapidly changing business landscape.
Challenges in Implementing Generative BI
While generative BI holds immense potential, its implementation poses several challenges that organizations must address:
Data Quality and Integration Issues
Ensuring data quality and consistency across disparate sources remains a significant challenge in generative BI initiatives. Poor data quality can lead to inaccurate insights and flawed decision-making, underscoring the importance of robust data governance and integration practices.
Skill Gap
Generative BI requires specialized skills in data science, machine learning, and advanced analytics, posing challenges for organizations lacking the requisite expertise. Bridging the skill gap through training and talent acquisition is essential to harness the full potential of generative BI.
Security Concerns
With the proliferation of data breaches and cyber threats, security remains a paramount concern in generative BI implementations. Protecting sensitive data and ensuring compliance with regulatory requirements demand robust security measures and proactive risk management strategies.
Case Studies Highlighting Generative BI Success
- Retail Optimization: A major retail chain implemented generative BI to enhance their inventory management and pricing strategies. By analyzing historical sales data and external factors such as weather patterns and economic indicators, the system generated predictive models to forecast demand for various products in real-time. This enabled the retailer to optimize inventory levels, adjust pricing dynamically based on demand fluctuations, and minimize stockouts and overstock situations. As a result, the retailer experienced a significant increase in sales, improved customer satisfaction, and enhanced profitability.
- Healthcare Predictive Analytics: A leading healthcare provider leveraged generative BI to improve patient outcomes and operational efficiency. By analyzing electronic health records, patient demographics, and clinical data, the system generated predictive models to identify patients at risk of developing chronic conditions or complications. This allowed healthcare providers to intervene proactively, implement preventive measures, and personalize treatment plans for high-risk patients. The implementation of generative BI resulted in reduced hospital readmissions, improved patient satisfaction, and lowered healthcare costs.
- Financial Services Fraud Detection: A multinational financial services company utilized generative BI to combat fraud and financial crimes. By analyzing transactional data, customer behavior patterns, and historical fraud cases, the system generated predictive models to detect suspicious activities and potential fraud incidents in real-time. This enabled the company to identify fraudulent transactions, block unauthorized access, and mitigate financial losses effectively. As a result, the company experienced a significant reduction in fraud-related losses, improved regulatory compliance, and enhanced customer trust and loyalty.
These case studies illustrate the successful implementation of generative BI across various industries, highlighting its ability to generate actionable insights, optimize decision-making processes, and drive tangible business outcomes.
Future Trends and Innovations in Generative BI
Future trends and innovations in Generative Business Intelligence (BI) are poised to further revolutionize the way organizations harness data for decision-making and strategic planning. Several key developments are anticipated:
- Advanced Predictive Modeling: Future advancements in generative BI will likely focus on refining predictive modeling techniques, leveraging deep learning algorithms and neural networks to extract deeper insights from data. These models will be capable of identifying complex patterns and relationships in large and diverse data sets, enabling organizations to make more accurate predictions and forecasts.
- Real-time Generative Insights: As technology continues to evolve, there will be a shift towards real-time generative insights, allowing organizations to analyze and act upon data as it is generated. This will enable quicker decision-making, enhanced situational awareness, and improved responsiveness to rapidly changing business conditions.
- Explainable AI: With the increasing adoption of AI-driven generative BI, there will be a growing emphasis on explainable AI techniques that provide transparency into how algorithms arrive at their conclusions. This will be crucial for building trust and confidence in AI-powered decision-making processes, particularly in regulated industries such as healthcare and finance.
- Augmented Analytics: Future innovations in generative BI will incorporate augmented analytics capabilities, combining machine learning with natural language processing and automation to enhance data exploration and interpretation. This will enable users to interact with data more intuitively, uncover hidden insights, and derive actionable recommendations more efficiently.
- Integration with IoT and Edge Computing: The integration of generative BI with Internet of Things (IoT) devices and edge computing technologies will enable organizations to leverage real-time sensor data for predictive analytics and decision-making. This convergence will facilitate the development of innovative applications in areas such as predictive maintenance, supply chain optimization, and smart manufacturing.
- Ethical and Responsible AI: As generative BI becomes more pervasive, there will be a heightened focus on ethical and responsible AI practices. Organizations will need to prioritize fairness, accountability, and transparency in their AI-driven decision-making processes, ensuring that biases are identified and mitigated, and that algorithms are used ethically and responsibly.
Overall, the future of generative BI holds tremendous potential for driving innovation, improving decision-making, and unlocking new opportunities for organizations across diverse industries. By embracing emerging trends and innovations, organizations can harness the full power of data to gain a competitive edge and thrive in an increasingly complex and dynamic business environment.
Conclusion
Generative Business Intelligence represents a paradigm shift in the realm of data analytics, offering predictive insights and actionable recommendations to drive business growth and innovation. By harnessing the power of machine learning, predictive analytics, and real-time data integration, organizations can unlock new opportunities, mitigate risks, and stay ahead of the competition in today’s dynamic marketplace.
FAQs
1. What distinguishes generative BI from traditional BI?
Generative BI goes beyond descriptive analytics to offer predictive and prescriptive insights, leveraging advanced algorithms and machine learning models.
2. How does generative BI benefit organizations?
Generative BI enhances decision-making, provides real-time insights, and offers scalability and flexibility to adapt to evolving business needs.
3. What challenges are associated with implementing generative BI?
Common challenges include data quality issues, skill gaps in data science, and security concerns related to data privacy and compliance.